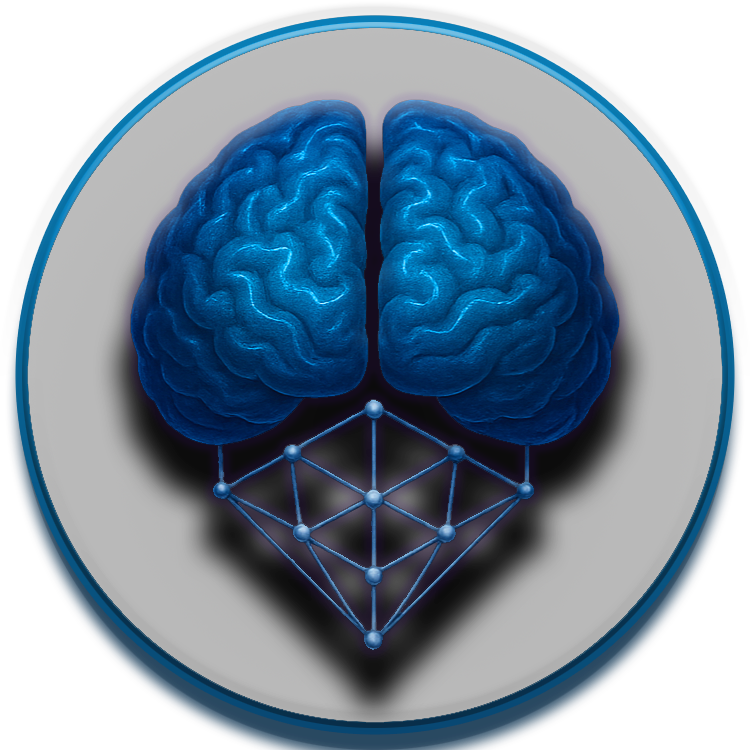
NeuroCognitive Architecture (NCA) Integration Guide¶
Table of Contents¶
- Introduction
- Integration Overview
- Prerequisites
- Installation
- API Integration
- SDK Integration
- Memory System Integration
- LLM Integration
- Configuration Options
- Authentication and Security
- Monitoring and Observability
- Troubleshooting
- Best Practices
- Examples
- FAQ
- Support
Introduction¶
This guide provides comprehensive instructions for integrating the NeuroCognitive Architecture (NCA) with your existing systems. NCA offers a biologically-inspired cognitive framework for Large Language Models (LLMs), featuring a three-tiered memory system, health dynamics, and advanced cognitive components that enhance LLM capabilities.
Integration Overview¶
The NCA can be integrated with your systems in several ways:
- REST API: For web applications and services that need to communicate with NCA over HTTP
- Python SDK: For direct integration in Python applications
- Docker Containers: For containerized deployment
- Kubernetes: For orchestrated deployment in cloud environments
Choose the integration method that best suits your technical requirements and infrastructure.
Prerequisites¶
Before integrating NCA, ensure you have:
- Python 3.9 or higher
- Docker (for containerized deployment)
- Kubernetes (for orchestrated deployment)
- Access to an LLM API (OpenAI, Anthropic, etc.)
- Database system (PostgreSQL recommended)
- Redis (for caching and pub/sub)
- 8GB+ RAM for development, 16GB+ for production
Installation¶
Using pip¶
Using Docker¶
docker pull neuroca/neuroca:latest
docker run -p 8000:8000 -e API_KEY=your_api_key neuroca/neuroca:latest
From Source¶
API Integration¶
The NCA exposes a RESTful API that allows you to interact with the system programmatically.
API Authentication¶
All API requests require authentication using an API key:
curl -X POST https://api.neuroca.dev/v1/cognitive/process \
-H "Authorization: Bearer YOUR_API_KEY" \
-H "Content-Type: application/json" \
-d '{"input": "Your input text here"}'
Core API Endpoints¶
Endpoint | Method | Description |
---|---|---|
/v1/cognitive/process | POST | Process input through the cognitive pipeline |
/v1/memory/store | POST | Store information in memory |
/v1/memory/retrieve | GET | Retrieve information from memory |
/v1/health/status | GET | Get system health status |
/v1/config | GET/PUT | Get or update configuration |
API Response Format¶
All API responses follow a standard format:
{
"status": "success",
"data": {
// Response data here
},
"metadata": {
"request_id": "req_123456",
"processing_time": "0.235s"
}
}
SDK Integration¶
For Python applications, the NCA SDK provides a more direct integration experience.
Installation¶
Basic Usage¶
from neuroca import NeuroCognitiveArchitecture
# Initialize the NCA with your API key
nca = NeuroCognitiveArchitecture(api_key="your_api_key")
# Process input through the cognitive pipeline
response = nca.process("Your input text here")
# Access different memory tiers
working_memory = nca.memory.working.get()
episodic_memory = nca.memory.episodic.retrieve("query")
semantic_memory = nca.memory.semantic.query("concept")
# Monitor health
health_status = nca.health.status()
Memory System Integration¶
The NCA's three-tiered memory system can be integrated with your existing data storage solutions.
Working Memory Integration¶
Working memory is designed for short-term, high-speed access:
# Store in working memory
nca.memory.working.store(key="user_context", value={"user_id": "123", "session": "active"})
# Retrieve from working memory
context = nca.memory.working.get("user_context")
Episodic Memory Integration¶
Episodic memory stores experiences and events:
# Store an episode
nca.memory.episodic.store(
content="User completed signup process",
metadata={"timestamp": "2023-06-15T14:30:00Z", "user_id": "123"}
)
# Retrieve relevant episodes
episodes = nca.memory.episodic.retrieve("signup process")
Semantic Memory Integration¶
Semantic memory stores concepts and knowledge:
# Store semantic information
nca.memory.semantic.store(
concept="customer_preferences",
data={"likes": ["fast response", "clear explanations"], "dislikes": ["technical jargon"]}
)
# Query semantic memory
preferences = nca.memory.semantic.query("customer_preferences")
LLM Integration¶
NCA is designed to work with various LLM providers:
OpenAI Integration¶
from neuroca.integration.llm import OpenAIProvider
# Configure OpenAI as the LLM provider
nca.configure_llm(
provider=OpenAIProvider(
api_key="your_openai_api_key",
model="gpt-4"
)
)
Anthropic Integration¶
from neuroca.integration.llm import AnthropicProvider
# Configure Anthropic as the LLM provider
nca.configure_llm(
provider=AnthropicProvider(
api_key="your_anthropic_api_key",
model="claude-2"
)
)
Custom LLM Integration¶
You can integrate custom LLMs by implementing the LLMProvider
interface:
from neuroca.integration.llm import LLMProvider
class CustomLLMProvider(LLMProvider):
def __init__(self, **kwargs):
super().__init__(**kwargs)
# Initialize your custom LLM here
def generate(self, prompt, **params):
# Implement generation logic
pass
def embed(self, text):
# Implement embedding logic
pass
# Configure custom LLM provider
nca.configure_llm(provider=CustomLLMProvider())
Configuration Options¶
NCA offers extensive configuration options to customize its behavior:
nca.configure({
"memory": {
"working": {
"capacity": 10,
"ttl": 3600 # Time to live in seconds
},
"episodic": {
"storage_backend": "postgres",
"retrieval_strategy": "semantic_search"
},
"semantic": {
"embedding_model": "text-embedding-ada-002",
"vector_db": "pinecone"
}
},
"health": {
"monitoring_interval": 60, # seconds
"alerting_threshold": 0.8
},
"cognitive": {
"attention_mechanism": "priority_based",
"reasoning_depth": "medium"
}
})
Authentication and Security¶
API Key Management¶
Generate and manage API keys through the NCA dashboard or API:
# Generate a new API key
curl -X POST https://api.neuroca.dev/v1/auth/keys \
-H "Authorization: Bearer ADMIN_API_KEY" \
-H "Content-Type: application/json" \
-d '{"name": "Production API Key", "permissions": ["read", "write"]}'
Data Encryption¶
NCA encrypts sensitive data at rest and in transit. Configure encryption settings:
nca.configure_security({
"encryption": {
"at_rest": True,
"key_rotation_days": 90,
"algorithm": "AES-256-GCM"
}
})
Monitoring and Observability¶
Health Metrics¶
Monitor NCA's health metrics:
# Get current health metrics
health = nca.health.status()
# Subscribe to health events
def health_alert(event):
if event["level"] == "critical":
# Handle critical health event
pass
nca.health.subscribe(health_alert)
Logging Integration¶
Integrate NCA logs with your logging system:
import logging
# Configure NCA logging
nca.configure_logging({
"level": "INFO",
"format": "%(asctime)s - %(name)s - %(levelname)s - %(message)s",
"handlers": ["console", "file"]
})
# Or use your existing logger
custom_logger = logging.getLogger("your_app")
nca.set_logger(custom_logger)
Troubleshooting¶
Common Issues¶
- Connection Errors
- Check network connectivity
- Verify API key is valid
-
Ensure firewall allows connections
-
Memory Retrieval Issues
- Verify data was properly stored
- Check query parameters
-
Ensure vector database is operational
-
LLM Integration Problems
- Verify LLM API key is valid
- Check rate limits
- Ensure prompt format is correct
Diagnostic Tools¶
# Run diagnostics
diagnostics = nca.diagnostics.run()
print(diagnostics.summary())
# Test specific components
memory_test = nca.diagnostics.test_component("memory")
llm_test = nca.diagnostics.test_component("llm")
Best Practices¶
- Memory Management
- Regularly clean up working memory
- Index important information in semantic memory
-
Store contextual experiences in episodic memory
-
Performance Optimization
- Use batch operations for multiple items
- Implement caching for frequent queries
-
Configure memory retrieval thresholds appropriately
-
Security
- Rotate API keys regularly
- Limit permissions to what's necessary
- Monitor for unusual access patterns
Examples¶
Conversational Agent Integration¶
from neuroca import NeuroCognitiveArchitecture
nca = NeuroCognitiveArchitecture(api_key="your_api_key")
def handle_user_message(user_id, message):
# Store user message in episodic memory
nca.memory.episodic.store(
content=message,
metadata={"user_id": user_id, "type": "incoming"}
)
# Process through cognitive pipeline
response = nca.process(
input=message,
context={"user_id": user_id}
)
# Store system response in episodic memory
nca.memory.episodic.store(
content=response.text,
metadata={"user_id": user_id, "type": "outgoing"}
)
return response.text
Knowledge Management System¶
from neuroca import NeuroCognitiveArchitecture
nca = NeuroCognitiveArchitecture(api_key="your_api_key")
def index_document(document):
# Extract key concepts
concepts = nca.cognitive.extract_concepts(document.text)
# Store in semantic memory
for concept in concepts:
nca.memory.semantic.store(
concept=concept.name,
data={"source": document.id, "context": concept.context}
)
# Store document in episodic memory
nca.memory.episodic.store(
content=document.text,
metadata={"document_id": document.id, "title": document.title}
)
return {"indexed_concepts": len(concepts)}
FAQ¶
General Questions¶
Q: Can NCA work offline?
A: NCA requires internet connectivity for LLM API calls, but can operate with reduced functionality using local components only.
Q: How does NCA handle rate limits from LLM providers?
A: NCA implements automatic retry mechanisms with exponential backoff and can be configured to switch between providers if rate limits are reached.
Q: Is NCA suitable for real-time applications?
A: Yes, NCA is designed for real-time applications with configurable performance parameters to balance response time and quality.
Technical Questions¶
Q: How does NCA handle large volumes of memory?
A: NCA uses vector databases for efficient storage and retrieval, with automatic sharding and indexing for large datasets.
Q: Can I use multiple LLM providers simultaneously?
A: Yes, NCA supports configuring multiple LLM providers with routing rules based on task type, cost, or performance requirements.
Q: How secure is the data stored in NCA?
A: NCA implements encryption at rest and in transit, with configurable data retention policies and access controls.
Support¶
For additional support:
- Documentation: https://docs.neuroca.dev
- GitHub Issues: https://github.com/neuroca/neuroca/issues
- Community Forum: https://community.neuroca.dev
- Email Support: justin@neuroca.dev
For enterprise support options, please contact justin@neuroca.dev.
This documentation is subject to updates. Last updated: 2023-10-15